On-field performance test and calibration of two commercially available low-cost sensors devices for CO2 monitoring
Price
Free (open access)
Volume
Volume 5 (2022), Issue 1
Pages
7
Page Range
15 - 22
Paper DOI
10.2495/EI-V5-N1-15-22
Copyright
WIT Press
Author(s)
H. Chojer, P.T.B.S. Branco, F.G. Martins & S.I.V. Sousa
Abstract
The use of low-cost devices for air quality monitoring is rapidly growing, and the reason behind the growth might (at least partially) be the real-time monitoring at a lower fixed and operating cost, ease of use and portability. nevertheless, the poor data reliability of low-cost sensors (LCS) remains a considerable challenge, especially when deployed in real-world conditions. This study aimed to evaluate and improve the performance of two commercially available indoor air quality monitoring LCS devices: AirVisual Pro and uRAD Monitor A3 (uRAD), which were used to monitor CO2 via non-dispersive infrared technology. The analysis took place from June to July 2019 in several classrooms of an urban school in Porto city. Machine learning techniques such as multivariate linear, support vector, gradient boosting and XGBoost regression models were used to perform an on-field calibration for improving the data accuracy of the devices. The results showed that although both the devices showed a strong linear correlation (r > 0.9) with the reference device, they might indicate deviated CO2 concentrations if used in their advertised plug and play format. Specifically, uRAD showed a steady offset compared to the reference values, while AirVisual Pro showed lower deviations than uRAD. The on-field calibration models improved the reliability and showed low root mean square error values (around 30 mg/m3) and a high coefficient of determination (0.99).
Keywords
carbon dioxide, low-cost sensors, machine learning
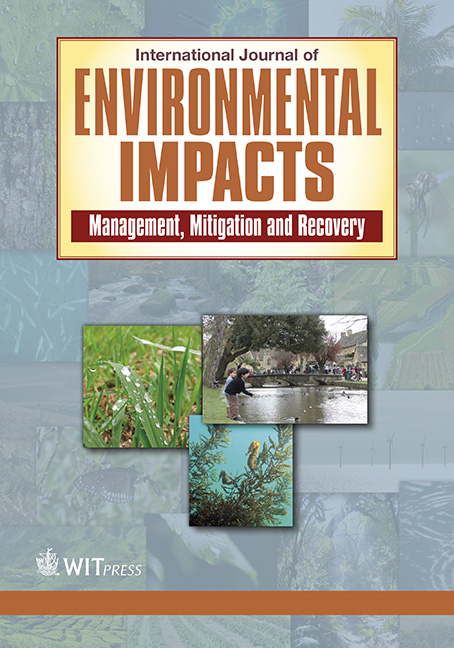