Recognition of track defects through measured acceleration using a recurrent neural network
Price
Free (open access)
Volume
Volume 8 (2020), Issue 3
Pages
10
Page Range
270 - 280
Paper DOI
10.2495/CMEM-V8-N3-270-280
Copyright
WIT Press
Author(s)
Sebastian Bahamon-Blanco, Sebastian Rapp, Yi Zhang, Jing Liu & Ullrich Martin
Abstract
As part of an optimized maintenance strategy, track monitoring should provide information to predict track faults at an early stage. This is possible by continuously measuring the axle box accelerations and using artificial intelligence, which can detect short wave defects on the railway track with high accuracy. Such short wave defects include rail breaks, cracks, and local irregularities (mud spots). These types of faults can reduce the track quality in a short period of time.
Different track irregularities were simulated in a track-vehicle scale model to generate acceleration data for typical track defects. The main focus of the current research is on recognition of local irregularities in the track-vehicle scale model. To implement the artificial intelligence, a Recurrent Neural Network is used to show the procedure and the results of recognition of track defects. The architecture and components of the neural network used are described in detail in this article. At the end of the article, a table summarizing the results of the different models trained for detecting the local irregularities in the track-vehicle scale model is presented.
Keywords
artificial intelligence, deep learning, detection, local instability, maintenance, railway.
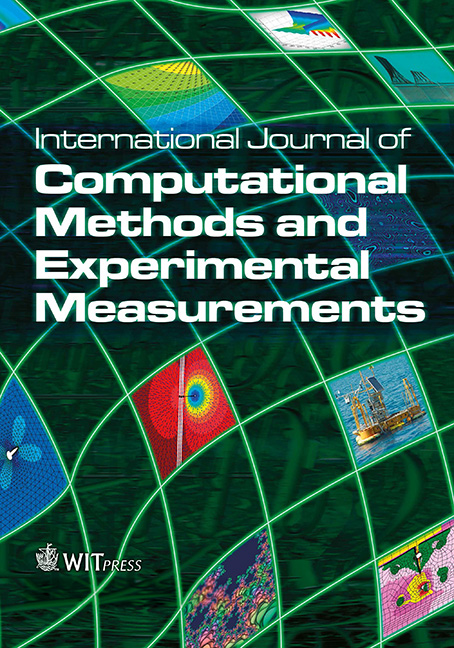