APPLICATION OF ARTIFICIAL NEURAL NETWORKS TO FORECASTING MONTHLY RAINFALL ONE YEAR IN ADVANCE FOR LOCATIONS WITHIN THE MURRAY DARLING BASIN, AUSTRALIA
Price
Free (open access)
Volume
Volume 12 (2017), Issue 8
Pages
16
Page Range
1282 - 1298
Paper DOI
10.2495/SDP-V12-N8-1282-1298
Copyright
WIT Press
Author(s)
JOHN ABBOT & JENNIFER MAROHASY
Abstract
Much of Australia regularly experiences extremes of drought and flooding, with high variability in rainfall in many regions of the continent. Development of reliable and accurate medium-term rainfall forecasts is important, particularly for agriculture. Monthly rainfall forecasts 12 months in advance were made with artificial neural networks (ANNs), a form of artificial intelligence, for the locations of Bathurst Deniliquin, and Miles, which are agricultural hubs in the Murray Darling Basin, in south-eastern Australia. Two different approaches were used for the optimisation of the ANN models. In the first, all months in each calendar year were optimised together, while in the second approach, rainfall forecasts for each month of the year were made individually. For each of the three locations for most months, higher forecast skill scores were achieved using single-month optimizations. In the case of Bathurst, however, for the months of November and December, the root mean square error (RMSE) for all-month optimisation was lower than for single-month optimisation. The best overall rainfall forecasts for each site were obtained by generating a composite of the two approaches, selecting the forecast for each month with the lowest forecast errors. Composite model skill score levels of at least 40% above that of climatology were achieved for all three locations, whereas skill level derived from forecasts using general circulation models is generally only comparable to climatology at the long-lead time of 8 months.
Keywords
Artificial neural network, flooding, forecast, Murray Darling Basin, rainfall, skill score
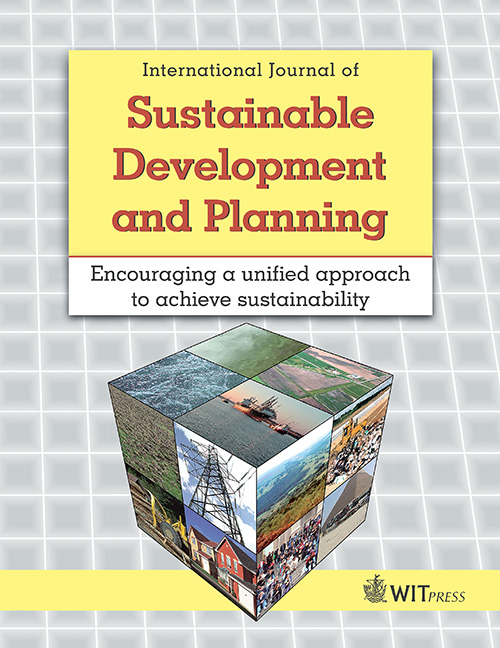