An ensemble clustering for mining high-dimensional biological big data
Price
Free (open access)
Volume
Volume 11 (2016), Issue 3
Pages
9
Page Range
328 - 337
Paper DOI
10.2495/DNE-V11-N3-328-337
Copyright
WIT Press
Author(s)
DEWAN MD. FARID, ANN NOWE & BERNARD MANDERICK
Abstract
Clustering of high-dimensional biological big data is incredibly difficult and challenging task, as the data space is often too big and too messy. The conventional clustering methods can be inefficient and ineffective on high-dimensional biological big data, because traditional distance measures may be dominated by the noise in many dimensions. An additional challenge in biological big data is that we need to find not only the clusters of instances (genes), but also for each cluster a set of features (conditions) that manifest the cluster. In this paper, we propose an ensemble clustering approach with feature selection and grouping for clustering high-dimensional biological big data. It uses two well-approved clustering methods: (a) k-means clustering and (b) similarity-based clustering. This approach selects the most relevant features in the dataset and grouping them into subset of features to overcome the problems associated with the traditional clustering methods. Also, we applied biclustering on each cluster that generated by ensemble clustering to find the sub-matrices in the biological data by the mean squared residue scores. We have applied the proposed clustering method on unlabeled genomic data (148 Exome datasets) of Brugada syndrome to discover previously unknown data pat- terns. Experiments verify that the proposed clustering method achieved high performance clustering results on high-dimensional biological big data.
Keywords
biclustering, biological big data, brugada syndrome, clustering, high-dimensional data
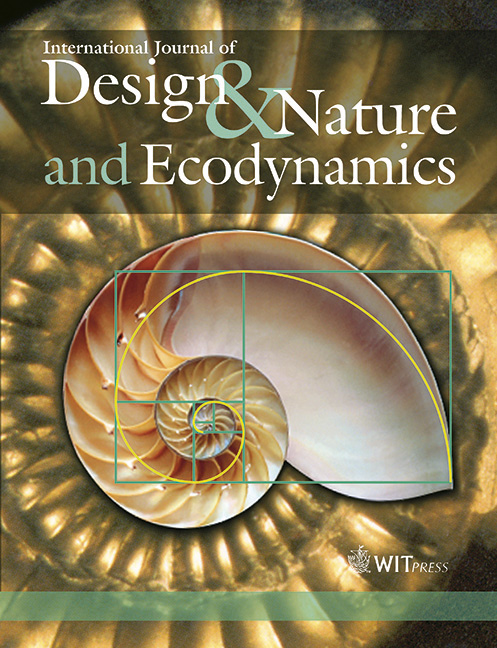