Reducing test time for selective populations in semiconductor manufacturing
Price
Free (open access)
Volume
Volume 11 (2016), Issue 3
Pages
9
Page Range
258 - 267
Paper DOI
10.2495/DNE-V11-N3-258-267
Copyright
WIT Press
Author(s)
D. PARK, M. SCHULDENFREI & G. LEVY
Abstract
As the semiconductor industry prepares for the Internet of Things, one of the major challenges it will face is to maintain quality levels as the volume of devices continues to grow. Semiconductor devices are moving from items of convenience (PCs) to necessity (smartphones) to mission-critical (autonomous automobiles). One aspect of manufacturing operations that can, and must change, in the face of ever-tightening quality requirements is how to test the devices that are shipped into the end market more efficiently while maintaining very high levels of quality. One of the ways to achieve these diametrically opposed goals is through the use of Big Data analytics. Semiconductor manufacturing test today is a ‘one size fits all’ process, with every device being made to go through the same battery of tests. Devices that initially do not pass are retested to be sure they are not bad, but what about the devices that are ‘exceptionally good’? Testing devices that are so ‘tight’ in their tolerances that statistically they will easily pass any remaining test intended to catch marginal devices is a waste of time and manufacturing resources. Using Big Data analytics within a manu- facturing environment can enable companies to establish a ‘Quality Index’ where every individual device can be ‘scored’ independently. If that device achieves a high-enough quality score, it can be ‘excused’ from any further testing to accelerate overall manufacturing throughput with zero impact on quality. This paper will show how semiconductor companies today are putting Big Data solutions in place to improve overall product quality.
Keywords
automobiles, manufacturing, quality, semiconductor, test.
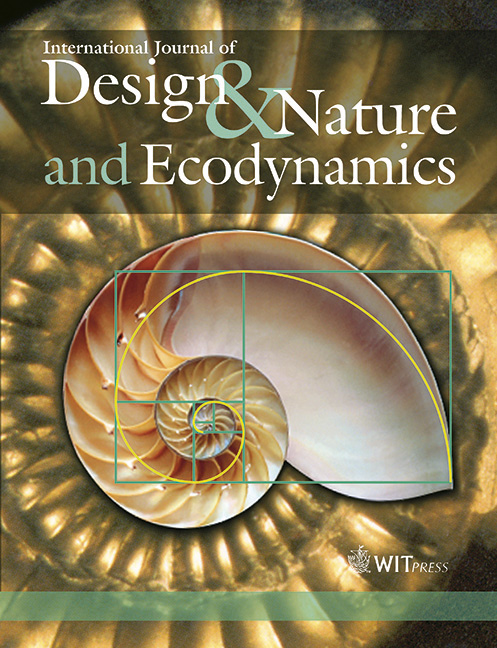